Schneider & Garg: Medical Researchers Find Cures by Conducting Many Studies and Failing Fast. We Need to Do the Same for Education
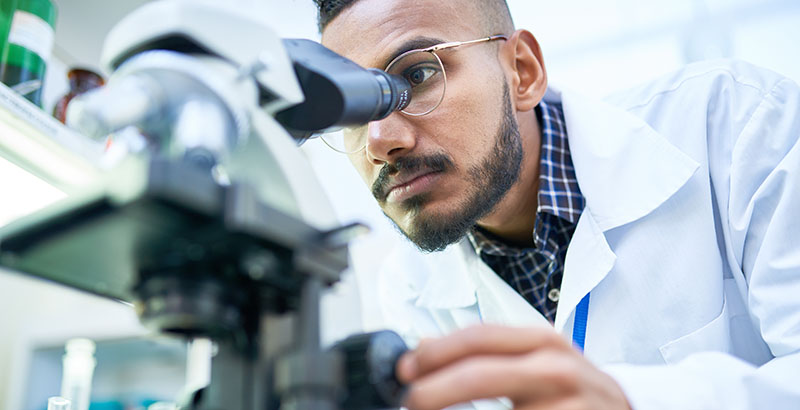
We start the 2020s with an uncomfortable truth: Even after decades of increasingly rigorous education research, what makes for effective learning is still unclear. Worse, the ability to effectively test what actually works is too limited and too slow.
As an example, consider the question of homework.
In math classrooms, the most common way to give homework is to assign a set of problems that let students practice what they were just taught. However, research from the learning sciences has shown that for middle school math students, mixing up problems of different types — involving concepts from earlier lessons as well as those just taught — leads to better learning.
But what does this finding mean for math homework for younger students or for homework in other content areas? And even within the context of middle school math, does mixing problems work for all students or only for those with a certain level of prior knowledge?
The learning sciences literature has no easy answer to these straightforward questions.
The reason: Experiments focused on small groups of learners will never be enough for answering the question of what works — especially when context and variation in prior knowledge, skills, aptitude and engagement drive so much of student outcomes.
More broadly, there needs to be far more learning about learning to improve outcomes for all students. The country’s education system should take advantage of technology to learn faster, learn at scale and then deploy those insights to benefit children.
The two of us have worked for different presidential administrations, and there are many areas where we disagree. But we firmly believe that technology can play a critical role in dramatically improving the research on learning.
Learning research should become much faster and larger in scale by leveraging the rapid growth of digital learning platforms, like Algebra Nation, which have thousands of users and can produce research at a much quicker pace than studies conducted solely in brick-and-mortar schools or classrooms. This increase could help researchers better understand when and where learning works for students.
That is important because a typical government-sponsored education research study can last five years, cost millions of dollars and often yield negative results. This is not an education-specific problem; low success rates are found in every science, from medicine to psychology.
But here’s a big difference: In the search for a drug to cure a disease such as Alzheimer’s, dozens of companies will conduct lots of different research studies. Most will fail, but if researchers are lucky, a handful of experiments will succeed and move on to an even more rigorous stage. In the end, hundreds of trials will yield effective medicine that addresses the disease.
The U.S. Department of Education’s Institute of Education Sciences is one of the only major investors in rigorous education research. The scope and time frame make for very slow progress, even with the millions of dollars it provides. In contrast to medical research, education doesn’t yet have dozens of companies investing in rigorous studies to discover what works.
The rise of large-scale ed tech platforms can help lead the way by allowing for more studies and encouraging the process of failing fast. With such additional research, educators will hopefully glean insights about what works for whom. It’s a model that demands our attention.
We have an opportunity to not only build out platforms for research but also attract the next wave of computer and data science talent and steer them toward the learning sciences and education, tapping their passion and the growing array of new methods.
This change is happening across the sciences. Starting more than two decades ago with the Human Genome Project, a new generation of researchers began applying a data-centric and computing approach to biology, sparking the rapid growth of computational biology — with a burgeoning array of new therapies and scientific discoveries as a result.
The science of learning needs a similar transformation, and the field would benefit from what we call learning engineering. A new and growing area, learning engineering aims to harness the increasing availability of data to continuously test and refine our understanding of how children develop skills and knowledge, and to systematically design educational environments to build and apply these insights.
Learning engineers are not just using program data at the school or system level to see if a group of students is at risk of dropping out or going on to college, which is important. They are also employing very detailed data to inform how kids learn best in the schools, classrooms and communities in which they live and learn. For instance, we can now see if a failing student is rushing through an exam without closely reading the questions, or lacks the core knowledge to understand the questions in the first place.
We can also use technology to broaden who gets to do learning sciences research. We believe that many more classroom practitioners should be engaging in this endeavor. Technology can help by making it easier for teachers to launch high-quality studies, and new programs like Mathematica’s RCE Coach have simplified the running of a high-quality research project so that classroom educators can engage in the scientific process. As Dr. Melina Uncapher of the NewSchools Venture Fund has noted, the field needs a strategy for “inclusive R&D” in which practitioners are involved in designing solutions.
Of course, many issues still need to be addressed. Among them is privacy. With an ever-growing body of data, maintaining student privacy is critical, especially when data from multiple sources are merged. While providing researchers with the necessary information, data systems must protect privacy.
Transparency is equally important. In the sciences, there are growing concerns about the quality of research, and leaders in the field need to build a culture of sharing results and the underlying data that fed them. Addressing the balance between privacy and transparency demands that policymakers, computer and learning scientists, and school system administrators work together.
We know that what we are proposing is a big task, but it is also a big opportunity. Our two organizations are planning a series of meetings and other activities to explore new investments in using platforms for rigorously testing interventions to find what works and for whom.
If we can harness the combined power of learning sciences and computer science, we can usher in a new era of human development. Our children, our parents, our educators and our nation deserve no less.
Mark Schneider is director of the Institute of Education Sciences at the United States Department of Education. Before assuming that role, he was a vice president and Institute Fellow at the American Institutes for Research and the president of College Measures. He previously served as the U.S. commissioner of education statistics from 2005 to 2008 and is a distinguished professor emeritus of political science at the State University of New York, Stony Brook. Kumar Garg is senior director for technology and society at Schmidt Futures and a former adviser to the White House Office of Science and Technology Policy under President Barack Obama.
Get stories like these delivered straight to your inbox. Sign up for The 74 Newsletter